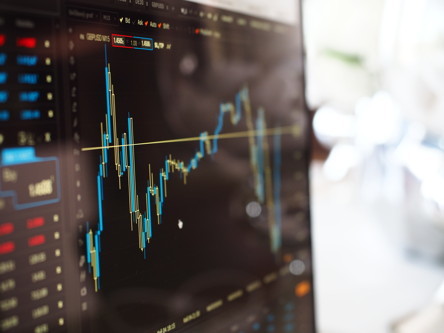
The data analysis and business intelligence (BI) market is one of the fastest-growing in the world, and e-commerce is one of the areas that can benefit most from these tools.
On the subject of numbers, International Data Corporation (IDC) reports indicate that Big Data and corporate analysis software revenues will rise from US$122 billion in 2015 to more than US$187 billion in 2019. In 5 years, they will have grown by more than 50%.
To be a bit more specific, the forecast for predictive analysis tools is that their revenues, worldwide, will jump from US$415 million, in 2014, to US$1.1 billion in 2019, giving a CAGR of 22%. Added to this, the number of organizations using some form of predictive analysis is expected to rise from 10% to 35% during the same period. The research is the work of Gartner, and is published in a report by Forbes.
All this growth is the effect of clear trends, such as a steadily increasing volume of available data, a growing interest in using it to manage businesses and the availability of faster computers and simpler software to process this huge load of data.
So if now it is easier to collect, process and analyze this data, retailers will find it easier to make use of it for a range of purposes in their business, such as marketing, stock levels and, most of all, their decision-making processes.
Before we go into these issues more thoroughly, we must first get a better understanding of how predictive analysis works.
How does predictive analysis work?
This type of analysis is based on past records and statistical algorithms for an improved forecast of future scenarios with the least margin of error possible.
It is a process that also uses machine learning to discover patterns, create rules and combine data from a number of sources and time series to get a better idea of what may happen in the future.
In practice, predictive analysis is able to spot trends, forecast behavior, project results of business indicators and support decision-making.
Big Data as a basis for predictive analysis
But to make the future more predictable, predictive analysis needs a large amount of high quality data. This is possible thanks to Big Data. Previously, the major challenges were to collect a greater amount of data and to attain the technical capacity to process it (both the hardware and the software for this were lacking).
Once an immense quantity of data, whether or not structured, can be handled, processed and cross-referenced with Big Data, predictive analysis becomes more efficient.
Now we have more than enough sources of information to be organized and used by companies, enabling them to optimize both the management and the operation of their business.
This has helped banking and credit card services, for example, to provide credit for users more efficiently based on their payment history and their purchasing behavior.
In the case of virtual stores, predictive analysis can be used to forecast annual revenues, identify a target audience for a marketing campaign and use systems for recommending products, among many other possibilities.
How can predictive analysis be applied in managing e-commerce?
Forecast of business indicators
Projecting a rise or a fall in revenues, billings or average ticket size can become a more precise exercise when based on predictive analysis.
Here, the key approach is to combine historical series of a particular indicator (billings, for example) with internal and external factors that can influence the forecast result.
Among these factors, we can mention the government’s financial and fiscal results (changes in GDP, the interest rate, inflation and other taxes), the financial data and performance of direct and indirect competitors, details of the company’s in-house projects, consumer data, etc.
Since there is a series of variables that are often unforeseeable, it is usual for an e-commerce manager to have more than one scenario (an ideal one and others that are conditional) at his disposal for analysis.
Indeed, the biggest challenge facing data analysts and scientists is to identify these potential interferences which are outside a store’s control. The more elements of this sort are detected, the fewer risks e-commerce managers will have to deal with.
Segmentation of marketing campaigns
In the case of marketing initiatives, predictive analysis can show the way to construct more personalized advertisements.
The approach used for this is to segment customers, i.e. details of consumers are extracted from an in-house database and cross-referenced with parameters defined by the marketing team to create more efficiently customized campaigns.
And here we are not talking about simple segmentation that can be done manually, such as separating people by gender, location or age. The idea is to detect patterns of behavior and use them to predict which content or products are most likely to be consumed by a specific target market.
For example, with the help of Big Data and predictive analysis, a fashion e-commerce business can create a campaign of sponsored posts on Facebook to advertise dresses to women who in the last three months have posted that they are going to be bridesmaids at a wedding. There is data for this – it just needs to be collected, processed and used for the correct purpose.
An interesting case for analysis and inspiration is Macy’s, which boosted online sales by between 8% and 12% within three months of installing a system to provide predictive analysis. The retailer used predictive models for a better segmentation of its email marketing campaigns.
Personalization in recommender systems
The use of predictive analysis in recommender and personalization systems is one of the most developed and best-known cases in the sector.
Its approach focuses on identifying the content and offers most likely to be taken up by the people who access the store’s website.
For this the recommender systems use predictive analysis to combine the historical series of customer purchases with their behavior and profiles.
Using this as a basis, various associations are made, such as:
- preferences of similar types of customer: shoppers in an online bookstore between 18 and 25 years of age, who buy books on history and philosophy, can create a behavioral pattern for purchases of the same books. So the system can indicate these specific works to any shopper with the same profile;
- reference by price: a customer who spends an average ticket of R$150.00 may be more likely to buy recommended products in this price range;
- combination of complementary products: a customer who buys a refrigerator and a stove in a short space of time may be furnishing a house, and so be interested in other household items such as a microwave oven, a set of saucepans or cutlery, and so on.
It should be noted that here the major challenge in predictive analysis is to discover the less obvious patterns in the profiles and behavior of customers. These are archetypes which you could not easily identify, such as associating the purchase of orange juice with sports stockings for women who run or who go to the gym every morning and need a source of vitamins to restore their energy.
Inventory management
The biggest challenge in inventory management is finding the minimum quantity of products needed to meet the demand from customers in a specific period of time.
To avoid both overstocking and understocking, an analysis of data on consumer behavior and historical series of sales may make the process more exact
For example, in the same way as a pattern of shopping behavior by one group of customers may determine the offers recommended on the store website, so may the same model be used to predict what products you should stock and in what quantities.
This approach can also use historical data and sales forecasts for a product to determine approximately the ideal quantity to stock.
Pricing products
On the subject of computerizing product pricing, many store owners opt for the more reactive analyses and solutions, such as A/B tests or changes in competitors’ prices.
However, you can also use predictive analysis for pricing, by taking a historical series of prices combined with sales forecasts or customer behavior (how people usually shop and what they spend on average).
In fact this is a complicated equation, since while a manual process is more vulnerable to error, automatic calculations can also damage a store’s margins if not monitored constantly.
Help in decision-making
One of the key functions of predictive analysis is to help a company make the right decision more frequently.
In e-commerce, store owners can use projections to identify, for example, the products that will sell most in a promotion, the public to be segmented for a marketing campaign or the ideal layout of a website, based on the browsing habits of shoppers.
However it should be noted that this type of analysis is not just predictive but also reactive. After all, there may be a bias in the owner’s mind towards a course of action, which may be changed or confirmed when an analyst submits a conclusion.
In fact, this is one of the problems of introducing predictive analysis which will be discussed in the next section.
What are the challenges to applying predictive analysis properly?
The growth we have referred to in the revenues of companies that use BI and predictive analysis shows that it has become fashionable to use tools of this type. Nowadays large companies are more prepared to use these systems, and many of them recognize that predictive analysis adds to business intelligence and, as a result, increases management efficiency.
One the other hand, there are many companies that say that they use predictive analysis only to add value to their brands, but they do not use these tools or do not use them correctly.
Specialist labor does not present too much of a challenge. Since the demand for predictive analysis solutions has grown in recent years, there are more and more qualified professionals in the market to do the work of collecting and analyzing data.
One of the problems may be in defining the data to be collected. Often an e-commerce company does not know what information it needs to extract (it has not set its objectives), and this can lead to too much or too little information being collected, processed and analyzed. This is a waste of time and money.
Moreover, many companies do not have the appropriate structure (hardware and software) to organize and analyze a large quantity of data. If this is the case, we recommend purchasing or outsourcing a full predictive analysis suite, such as SAS or Azure.
Another problem is the lack of communication between the person analyzing the data and the person making decisions based on the analysis. This is where guesswork comes in, and this perhaps is the biggest barrier to management based on data.
If an e-commerce manager, even with real and accurate projections in his hands, still bases decisions on his feelings, intuition or experience, then the use of predictive analysis tools makes little sense.
Conclusion
To sum up: forecasting the future has become a more exact science with the evolution of predictive analysis systems.
In e-commerce, this can represent an enormous gain in competitiveness and management intelligence. After all, it allows various business processes to be improved, such as segmentation for marketing purposes, inventory management and the pricing of offers.
But it must not be forgotten that the virtual store owner does not need to apply predictive analysis on every front. The rational outlook here is not simply to abandon guesswork, but also to know in which areas this analysis will have most effect.
In addition to predictive analysis, another trend making waves in e-commerce is the use of chatbots. In our article: Chatbots are Boosting Sales in E-Commerce, you can find out how these platforms are revolutionizing the way in which shoppers interact with virtual stores.